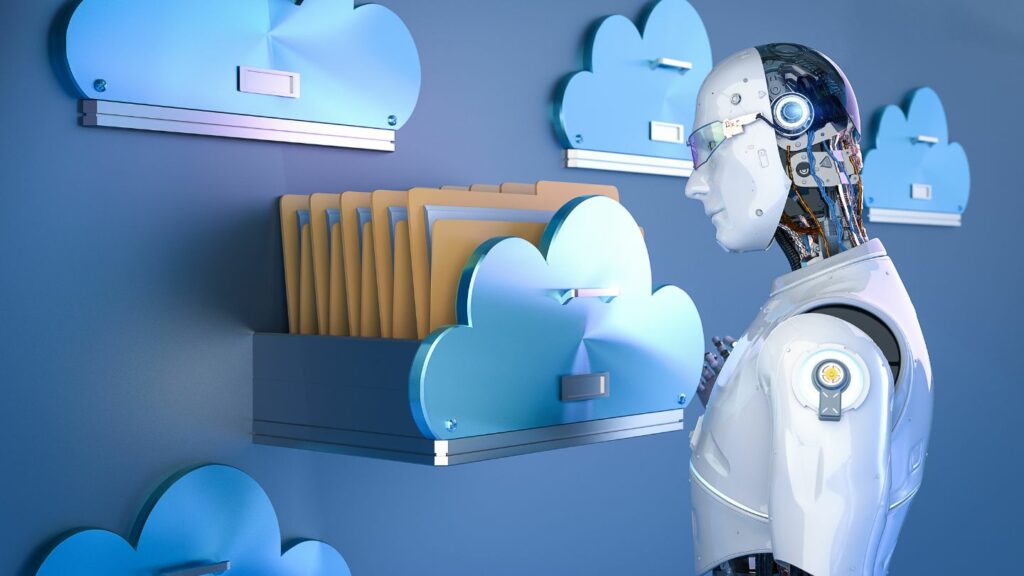
What is Predictive AI vs. Generative AI vs. Machine Learning?
In the world of Artificial Intelligence (AI), branches like Predictive AI, Generative AI, and Machine Learning (ML) have distinct roles. Their significance is particularly pronounced in the finance sector, where they reshape investment strategies, risk management, and customer engagement. Understanding these technologies is not just a tech endeavor; it’s fundamental to staying ahead in the fast-paced financial world.
Predictive AI
Predictive AI involves using historical data to forecast future trends or events. It’s a cornerstone in finance, aiding in risk management, market trend forecasting, and customer behavior analysis. Banks, for example, employ predictive AI for credit risk assessment, identifying potential loan defaulters. Insurance companies use it to predict claim risks, while investment firms leverage it for market trend analysis and portfolio management.
In finance, predictive AI has seen significant advancements. Trading bots in cryptocurrency and forex markets are prime examples, employing algorithms to detect trends and execute profitable trades. In stock trading, predictive AI helps in identifying market sentiments and investor behaviors, leading to more informed trading decisions.
Generative AI
Generative AI focuses on creating new content or data. While its use in finance is less extensive than predictive AI, its potential is noteworthy. For instance, it could automate financial report generation or simulate market scenarios for stress testing. Beyond finance, it finds applications in creating realistic simulations, generating creative content in advertising, and even assisting in product design by generating numerous iterations quickly.
Generative AI has made strides in content creation, such as realistic image and video generation, text, and even music. Its ability to analyze existing works and produce new creations has implications for creative industries, marketing, and even educational content development.

Machine Learning
Machine Learning, a fundamental AI aspect, involves algorithms learning and improving from data. In finance, ML algorithms are pivotal for pattern recognition in market data, fraud detection in transactions, and enhancing algorithmic trading strategies. They play a critical role in analyzing large datasets for insights that human analysts might miss, offering a more nuanced understanding of market dynamics and customer behavior.
ML’s role in finance has expanded, with firms like BlackRock using transformer-based LLMs for text analysis, improving investment decisions. These advancements signify a shift towards more nuanced and sophisticated financial analysis tools, enhancing the accuracy of predictions and the ability to manage complex portfolios.
Comparative Analysis
Purpose and Goals: Generative AI is about creation and innovation, producing new content and data. Predictive AI, conversely, is about forecasting and anticipation, leveraging past data to predict future trends. ML underlies both, providing the learning backbone for these technologies.
Applications in Finance: Predictive AI is more prevalent in finance, crucial for market analysis, risk management, and customer insights. Generative AI, while less applied, holds potential for automating content creation and scenario modeling. ML is the engine driving both, facilitating data analysis and pattern recognition.
Data and Model Requirements: Predictive AI demands high-quality historical data for accurate forecasting. Generative AI needs diverse datasets to generate realistic and varied outputs. ML hinges on the relevance and volume of data for effective learning.
In the finance industry, the distinction and understanding of Predictive AI, Generative AI, and ML are vital. Predictive AI leads in direct applications like market analysis and risk management. Generative AI, though less directly involved, offers potential for innovation and automation in financial reporting and market simulation. ML underpins both, advancing data analysis and decision-making processes. As these technologies evolve, they promise to further revolutionize the finance sector, offering new opportunities and necessitating a strategic approach to their adoption and implementation.
Future Prospects and Ethical Considerations
As AI continues to evolve, the finance sector must consider not only the technological advancements but also the ethical implications. Issues like data privacy, algorithmic bias, and the potential for job displacement are critical considerations. The future of finance with AI will involve navigating these challenges while leveraging the immense potential of these technologies for market analysis, customer engagement, and risk management.
Understanding and integrating these AI technologies are crucial for finance professionals. It’s not just about harnessing the power of AI but also about understanding its limitations and ethical considerations. As the landscape of finance continues to be reshaped by these technologies, professionals equipped with this knowledge will be better positioned to lead and innovate in this new era.
—
Sources:
1. BlackRock – How AI is Transforming Investing: https://www.blackrock.com/corporate/insights/blackrock-investment-institute/publications/ai-investing
2. Prompt Engineering Futures – AI in Finance Trends 2023: https://www.promptengineeringfutures.com/ai-in-finance-trends-2023-how-ai-changing-the-finance-industry
3. Talent500 Blog – Predictive analytics: Advancements in 2023 and their implications: https://talent500.co/blog/predictive-analytics-advancements-in-2023-and-their-implications